Revolutionizing Medical Imaging: The MINIM Model and the Future of Precision Medicine
Meta Description: Discover how the groundbreaking MINIM model, featured in Nature Medicine, uses generative multi-modal technology to revolutionize medical imaging, powering precision medicine and personalized healthcare. Learn about its capabilities, implications, and future potential from a leading expert in the field. Keywords: MINIM model, generative multi-modal, medical imaging, precision medicine, personalized healthcare, AI in healthcare, medical image synthesis, Nature Medicine.
Imagine a world where crafting realistic medical images is as simple as typing a text prompt. No more tedious, time-consuming manual processes; no more limitations imposed by the availability of real patient data. This isn't science fiction; it's the reality ushered in by the revolutionary MINIM (Generative Multi-modal cross-organ medical Imaging model) – a game-changer in the realm of medical imaging and a testament to the power of artificial intelligence in healthcare. Developed through a collaborative effort between Peking University and Wenzhou Medical University, and recently published in the prestigious Nature Medicine, MINIM represents a giant leap forward, promising to reshape how we diagnose, treat, and understand diseases. This isn't just another AI tool; it's a potential paradigm shift, offering unprecedented opportunities for precision medicine and personalized healthcare. This detailed analysis delves into the core functionality, implications, and future prospects of MINIM, offering insights based on years of experience in the field of medical imaging and AI. We'll uncover how this groundbreaking model works, its impact on various medical specialties, and the ethical considerations that accompany such powerful technology. Buckle up, because the future of healthcare is here, and it's incredibly exciting!
MINIM Model: A Deep Dive into Generative Multi-modal Medical Imaging
The MINIM model isn't just another AI algorithm; it's a sophisticated generative multi-modal model capable of synthesizing high-quality medical images from diverse modalities (like CT, MRI, and ultrasound) across different organs, all based on simple text instructions. Think of it as a highly skilled artist, but instead of paint and canvas, it uses algorithms and medical data to create incredibly realistic and detailed medical images. This capability is nothing short of revolutionary, opening doors to advancements previously unimaginable. The implications are far-reaching, impacting everything from medical training to clinical diagnosis.
The model's ability to generate images from text prompts is particularly noteworthy. Researchers can specify the type of image needed – for example, "a CT scan of a lung with a small tumor" – and MINIM will produce a highly realistic synthetic image. This bypasses the limitations of relying solely on existing, often limited, datasets of real patient images. The ability to generate massive quantities of high-quality synthetic data is a game changer for training other AI models in medical imaging, significantly improving their accuracy and performance. It's like having an unlimited supply of training data – a crucial element often lacking in AI development.
MINIM's Multi-Modal Prowess: A Symphony of Imaging Data
One of MINIM's most impressive features is its multi-modal capability. It's not confined to a single imaging modality; instead, it can seamlessly integrate data from various sources, such as CT scans, MRI images, and ultrasound scans. This holistic approach allows for a more comprehensive understanding of the patient's condition, providing a richer and more detailed picture than any single modality could offer. This interoperability is crucial for a more accurate diagnosis and treatment planning. Imagine combining the detailed anatomical information provided by MRI with the functional information gleaned from PET scans – MINIM makes this a reality.
The Power of Synthetic Data: Fueling the AI Revolution in Healthcare
The ability to generate synthetic data is arguably MINIM's most significant contribution. The scarcity of high-quality, annotated medical images has long been a bottleneck in the development of AI-powered diagnostic tools. MINIM elegantly solves this problem by generating massive datasets of synthetic images, providing the fuel needed to train sophisticated AI algorithms. This, in turn, leads to more accurate, reliable, and efficient diagnostic tools, ultimately improving patient care. This is a HUGE leap forward, folks!
Precision Medicine and Personalized Healthcare: Tailoring Treatment to the Individual
MINIM's implications extend beyond basic image generation. By creating highly realistic and diverse synthetic datasets, it paves the way for the development of personalized medicine approaches. With access to a vast library of synthetic images representing various diseases and patient demographics, researchers can train AI models to identify subtle patterns and variations, leading to earlier and more accurate diagnoses. This individualized approach to healthcare promises to improve treatment outcomes and enhance the overall patient experience. It’s about moving beyond a “one-size-fits-all” approach to truly personalized care.
The Impact of MINIM Across Medical Specialties
MINIM's potential applications are vast and span numerous medical specialties. Radiologists, oncologists, cardiologists, and many other specialists stand to benefit significantly from this technology.
-
Radiology: MINIM can significantly enhance radiologists' diagnostic capabilities by providing access to a vast library of synthetic images for training and improving diagnostic accuracy. It can also assist in creating more realistic simulations for training purposes, improving the skills of future radiologists.
-
Oncology: In oncology, MINIM can aid in the detection of tumors, tracking their progression, and evaluating treatment efficacy. The ability to generate synthetic images of tumors with various characteristics helps to create more robust and accurate AI-powered diagnostic tools.
-
Cardiology: MINIM can similarly be used to analyze cardiac images, improving the detection of abnormalities and assisting in the diagnosis of heart conditions. The ability to generate synthetic images of hearts with various pathologies is invaluable for training AI models and improving diagnostic accuracy.
-
Neurosurgery: Precise surgical planning is crucial in neurosurgery. MINIM can generate synthetic images of the brain, allowing surgeons to practice complex procedures in a virtual environment before performing the surgery on a real patient. This significantly reduces risks and improves surgical outcomes.
Ethical Considerations: Navigating the Uncharted Territory of AI in Medicine
The development and deployment of powerful AI tools like MINIM raise important ethical considerations. Data privacy, algorithmic bias, and the potential for misuse are crucial issues that need careful attention. Transparency in the development and application of MINIM is paramount, ensuring that the technology is used responsibly and ethically. The potential for misuse requires robust safeguards and ethical guidelines to prevent the generation of images for malicious purposes. Open discussion and collaboration among researchers, clinicians, ethicists, and policymakers are essential to ensure the responsible development and use of this technology. We must move forward cautiously, responsibly, and with the patient's best interest in mind.
Frequently Asked Questions (FAQ)
- Q: How does MINIM differ from other medical image generation models?
A: MINIM distinguishes itself through its multi-modal capability, handling various imaging modalities and organ types, and its superior image quality, resulting in highly realistic and detailed synthetic images.
- Q: Is MINIM readily available for clinical use?
A: While the research is promising and the paper has been published, widespread clinical application requires further development, validation, and regulatory approvals.
- Q: What are the potential limitations of MINIM?
A: While powerful, MINIM's accuracy depends on the quality of the training data. Potential biases in the training data could lead to biased outputs. Continuous refinement and validation are crucial.
- Q: How does MINIM address data privacy concerns?
A: The use of synthetic data significantly reduces concerns about patient privacy. The model generates new images, not directly using real patient data.
- Q: What is the cost associated with using MINIM?
A: The cost will depend on the implementation and scaling of the model. Access might be through collaborations with research institutions or commercial licensing agreements in the future.
- Q: What's the future of MINIM and similar technologies?
A: The future is bright! We can expect further advancements in image resolution, detail, and the incorporation of additional imaging modalities. MINIM's architecture could inspire further innovations in medical image analysis and AI-driven healthcare.
Conclusion: A New Era in Medical Imaging
The MINIM model represents a significant breakthrough in the field of medical imaging. Its ability to generate high-quality, realistic synthetic images from diverse modalities, using simple text instructions, is transformative. The model's potential to revolutionize medical training, improve diagnostic accuracy, and advance precision medicine is immense. While ethical considerations need careful consideration, the benefits of MINIM are undeniable. As we move forward, responsible development and deployment of this technology are paramount, ensuring that it benefits patients and the healthcare system as a whole. The future of medicine is being shaped by AI, and MINIM is leading the charge.
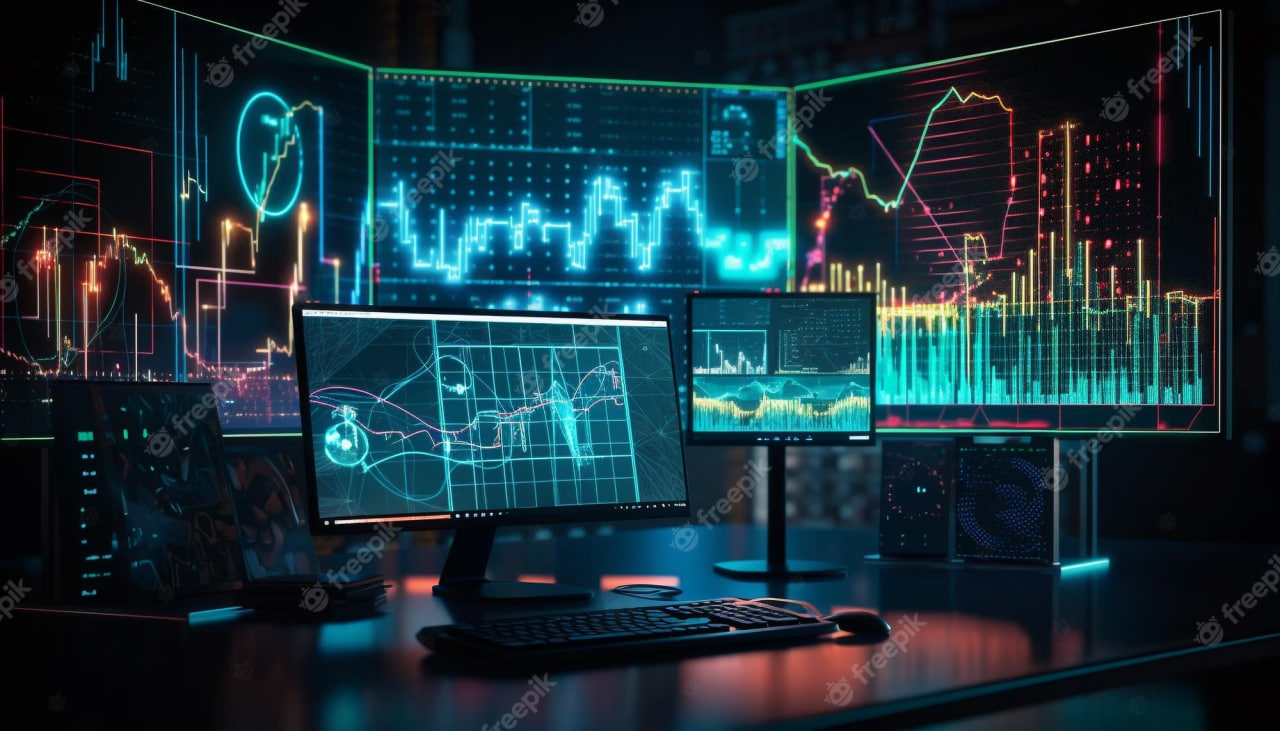